Дослідження системи NOMA на основі глибокого навчання з урахуванням мобільності вузлів та недосконалого CSI
DOI:
https://doi.org/10.20535/S0021347023070026Ключові слова:
частота символьних помилок, SER, стекова довга короткотривала пам’ять, S-LSTM, множинний вхід, множинний вихід, MIMO, неортогональний множинний доступ, глибока нейронна мережа, DNN, глибоке навчання, DL, інформація про стан каналу, CSIАнотація
В статті досліджується ефективність низхідної системи неортогонального множинного доступу NOMA (non-orthogonal multiple access) з використанням схеми стекової довгої короткотривалої пам’яті S-LSTM (stacked long short-term memory), що базується на глибокому навчанні DL (deep learning). Вважається, що канал зв’язку між автомобілями V2V (vehicle-to-vehicle) є селективним за часом внаслідок мобільності вузлів та наявності неточної інформації про стан каналу CSI (channel state information). Використання моделей незалежних та ідентично розподілених IID каналів (independent and identically distributed) з завмираннями п’ятого покоління (5G) на основі ліній затримки типу C TDL-C (tapped delay line type C) дозволяє створювати відгалужувачі каналів, які належним чином відтворюють бездротовий канал з завмираннями Nakagami-m. У статті досліджено ймовірність відключення OP (outage probability) та частоту символьних помилок SER (symbol error rate) як традиційних, так і запропонованих канальних оцінювачів. В статті проаналізовані ці характеристики при різних параметрах завмирання, пілотних символах PS (pilot symbols), швидкості навчання LR (learning rate) та розмірах пакетів. Навчання моделей глибоких нейронних мереж DNN (deep neural network) виконано за допомогою оптимізатора Adam. Підвищення відношення сигнал/шум SNR (signal-to-noise ratio) може зменшити SER, а отже, покращити ідентифікацію каналу низхідної лінії зв’язку в системах на основі стільникових мереж NOMA. Зменшення LR позитивно впливає на SER, підтверджуючи аналітичні висновки, які вказують на більші зміни у вагах DNN і більші помилки валідації при збільшенні LR. Проте ця перевага супроводжується недоліком частішого оновлення, що призводить до затримки збіжності моделі.
Посилання
Д. Бора, С. Баруах, С. Дас, Д. Бисвас, “Анализ работы 5G сотовых сетей Massive MIMO и малых сот: Моделирование,” Известия вузов. Радиоэлектроника, vol. 65, no. 6, pp. 341–351, 2022, doi: https://doi.org/10.20535/S0021347022060024.
Д. А. Василенко, “Природные алгоритмы оптимизации в задачах синтеза встроенных антенн IoT-устройств (обзор),” Известия вузов. Радиоэлектроника, vol. 65, no. 3, pp. 131–151, 2022, doi: https://doi.org/10.20535/S0021347022030013.
J. Merin Joshiba, D. Judson, V. Bhaskar, “A comprehensive review on NOMA assisted emerging techniques in 5G and beyond 5G wireless systems,” Wirel. Pers. Commun., vol. 130, no. 4, pp. 2385–2405, 2023, doi: https://doi.org/10.1007/s11277-023-10384-6.
R. Shankar et al., “Impact of node mobility on the DL based uplink and downlink MIMO-NOMA network,” Int. J. Inf. Technol., vol. 15, no. 6, pp. 3391–3404, 2023, doi: https://doi.org/10.1007/s41870-023-01362-z.
J. Alanya-Beltran, R. Shankar, P. Krishna, S. Kumar S, “Investigation of bi-directional LSTM deep learning-based ubiquitous MIMO uplink NOMA detection for military application considering robust channel conditions,” J. Def. Model. Simul. Appl. Methodol. Technol., vol. 20, no. 2, pp. 229–244, 2023, doi: https://doi.org/10.1177/15485129211050403.
T. Hirai, N. Wakamiya, T. Murase, “NOMA-dependent low-powered retransmission in sensing-based SPS for cellular-V2X mode 4,” in 2022 IEEE 96th Vehicular Technology Conference (VTC2022-Fall), 2022, pp. 1–7, doi: https://doi.org/10.1109/VTC2022-Fall57202.2022.10012826.
T. Hirai, “Non-orthogonal multiple access for infrastructure-less cellular-V2X,” IEICE Tech. Rep., vol. 122, no. 48, pp. 38–42, 2022, uri: https://ken.ieice.org/ken/paper/202205272CK5/eng/.
S. Patel, D. Chauhan, S. Gupta, “An overview of non orthogonal multiple access for future radio communication,” in 2021 International Conference on Intelligent Technologies (CONIT), 2021, pp. 1–3, doi: https://doi.org/10.1109/CONIT51480.2021.9498336.
G. Liu, Z. Wang, J. Hu, Z. Ding, P. Fan, “Cooperative NOMA broadcasting/multicasting for low-latency and high-reliability 5G cellular V2X communications,” IEEE Internet Things J., vol. 6, no. 5, pp. 7828–7838, 2019, doi: https://doi.org/10.1109/JIOT.2019.2908415.
A. Ihsan, W. Chen, S. Zhang, S. Xu, “Energy-efficient NOMA multicasting system for beyond 5G cellular V2X communications with imperfect CSI,” IEEE Trans. Intell. Transp. Syst., vol. 23, no. 8, pp. 10721–10735, 2022, doi: https://doi.org/10.1109/TITS.2021.3095437.
R. Shankar, V. V. R. Raman, K. P. Rane, B. K. Sarojini, R. Neware, “An investigation of the MIMO space time block code based selective decode and forward relaying network over n-u fading channel conditions,” J. Telecommunictions Inf. Technol., vol. 1, no. 2022, pp. 79–92, 2022, doi: https://doi.org/10.26636/jtit.2022.150421.
R. Shankar, P. Krishna, R. Naraiah, “Examination of the multiple-input multiple-output space-time block-code selective decode and forward relaying protocol over non-homogeneous fading channel conditions,” J. Def. Model. Simul. Appl. Methodol. Technol., vol. 20, no. 2, pp. 245–258, 2023, doi: https://doi.org/10.1177/15485129211047598.
L. Han, W.-P. Zhu, M. Lin, “Outage performance of downlink coordinated direct and relay transmission with NOMA over Nakagami-m fading channels,” in 2020 IEEE 92nd Vehicular Technology Conference (VTC2020-Fall), 2020, pp. 1–6, doi: https://doi.org/10.1109/VTC2020-Fall49728.2020.9348857.
N. Jaiswal, A. Pandey, S. Yadav, N. Purohit, L. Bariah, S. Muhaidat, “On the performance of NOMA-enabled V2V communications under joint impact of nodes mobility and channel estimation error,” in 2021 4th International Conference on Advanced Communication Technologies and Networking (CommNet), 2021, pp. 1–9, doi: https://doi.org/10.1109/CommNet52204.2021.9641915.
N. Jaiswal, A. Pandey, S. Yadav, N. Purohit, D. S. Gurjar, “Physical layer security performance of NOMA-aided vehicular communications over Nakagami-m time-selective fading channels with channel estimation errors,” IEEE Open J. Veh. Technol., vol. 4, pp. 72–100, 2023, doi: https://doi.org/10.1109/OJVT.2022.3222187.
Y. M. Khattabi, S. A. Alkhawaldeh, M. M. Matalgah, O. S. Badarneh, R. Mesleh, “Vehicle-to-roadside-unit-to-vehicle communication system under different amplify-and-forward relaying schemes,” Veh. Commun., vol. 38, p. 100539, 2022, doi: https://doi.org/10.1016/j.vehcom.2022.100539.
D.-T. Do, T. Anh Le, T. N. Nguyen, X. Li, K. M. Rabie, “Joint impacts of imperfect CSI and imperfect SIC in cognitive radio-assisted NOMA-V2X communications,” IEEE Access, vol. 8, pp. 128629–128645, 2020, doi: https://doi.org/10.1109/ACCESS.2020.3008788.
M. R. Mahmood, M. A. Matin, P. Sarigiannidis, S. K. Goudos, G. K. Karagiannidis, “Residual compensation-based extreme learning machine for MIMO-NOMA receiver,” IEEE Access, vol. 11, pp. 13398–13407, 2023, doi: https://doi.org/10.1109/ACCESS.2023.3242917.
A. Emir, F. Kara, H. Kaya, X. Li, “Deep learning-based flexible joint channel estimation and signal detection of multi-user OFDM-NOMA,” Phys. Commun., vol. 48, p. 101443, 2021, doi: https://doi.org/10.1016/j.phycom.2021.101443.
B. A. O, J. Surendran, “Joint channel estimation and signal detection in MIMO-NOMA wireless systems using deep learning,” in 2023 IEEE International Conference on Recent Advances in Systems Science and Engineering (RASSE), 2023, pp. 1–6, doi: https://doi.org/10.1109/RASSE60029.2023.10363503.
R. Shankar, B. K. Sarojini, H. Mehraj, A. S. Kumar, R. Neware, A. Singh Bist, “Impact of the learning rate and batch size on NOMA system using LSTM-based deep neural network,” J. Def. Model. Simul. Appl. Methodol. Technol., vol. 20, no. 2, pp. 259–268, 2023, doi: https://doi.org/10.1177/15485129211049782.
R. Shankar, M. K. Beuria, S. S. Singh, F. Ana, H. Mehraj, V. G. Krishnan, “5G NOMA defense application environment and stacked LSTM network architectures,” J. Mob. Multimed., 2022, doi: https://doi.org/10.13052/jmm1550-4646.1914.
C. Nguyen, T. M. Hoang, A. A. Cheema, “Channel estimation using CNN-LSTM in RIS-NOMA assisted 6G network,” IEEE Trans. Mach. Learn. Commun. Netw., vol. 1, pp. 43–60, 2023, doi: https://doi.org/10.1109/TMLCN.2023.3278232.
H. Huang, Y. Yang, Z. Ding, H. Wang, H. Sari, F. Adachi, “Deep learning-based sum data rate and energy efficiency optimization for MIMO-NOMA systems,” IEEE Trans. Wirel. Commun., vol. 19, no. 8, pp. 5373–5388, 2020, doi: https://doi.org/10.1109/TWC.2020.2992786.
X. Wang, P. Zhu, D. Li, Y. Xu, X. You, “Pilot-assisted SIMO-NOMA signal detection with learnable successive interference cancellation,” IEEE Commun. Lett., vol. 25, no. 7, pp. 2385–2389, 2021, doi: https://doi.org/10.1109/LCOMM.2021.3070705.
A. Emir, F. Kara, H. Kaya, H. Yanikomeroglu, “Deep learning empowered semi-blind joint detection in cooperative NOMA,” IEEE Access, vol. 9, pp. 61832–61852, 2021, doi: https://doi.org/10.1109/ACCESS.2021.3074350.
H. Ye, G. Y. Li, B.-H. Juang, “Power of deep learning for channel estimation and signal detection in OFDM systems,” IEEE Wirel. Commun. Lett., vol. 7, no. 1, pp. 114–117, 2018, doi: https://doi.org/10.1109/LWC.2017.2757490.
D. K. Patel et al., “Performance analysis of NOMA in vehicular communications over i.n.i.d Nakagami-m fading channels,” IEEE Trans. Wirel. Commun., vol. 20, no. 10, pp. 6254–6268, 2021, doi: https://doi.org/10.1109/TWC.2021.3073050.
M. Gaballa, M. Abbod, A. Aldallal, “Investigating the combination of deep learning for channel estimation and power optimization in a non-orthogonal multiple access system,” Sensors, vol. 22, no. 10, p. 3666, 2022, doi: https://doi.org/10.3390/s22103666.
M. H. Rahman, M. A. S. Sejan, M. A. Aziz, Y.-H. You, H.-K. Song, “HyDNN: A hybrid deep learning framework based multiuser uplink channel estimation and signal detection for NOMA-OFDM system,” IEEE Access, vol. 11, pp. 66742–66755, 2023, doi: https://doi.org/10.1109/ACCESS.2023.3290217.
Z. Tang, J. Wang, J. Wang, J. Song, “On the achievable rate region of NOMA under outage probability constraints,” IEEE Commun. Lett., vol. 23, no. 2, pp. 370–373, 2019, doi: https://doi.org/10.1109/LCOMM.2018.2870584.
S. Li, M. Derakhshani, S. Lambotharan, “Outage-constrained robust power allocation for downlink MC-NOMA with imperfect SIC,” in 2018 IEEE International Conference on Communications (ICC), 2018, pp. 1–7, doi: https://doi.org/10.1109/ICC.2018.8422364.
M. Gaballa, M. Abbod, A. Jameel, “Power optimization analysis using throughput maximization in MISO non-orthogonal multiple access system,” in 2021 IEEE Globecom Workshops (GC Wkshps), 2021, pp. 1–6, doi: https://doi.org/10.1109/GCWkshps52748.2021.9682080.
M. Gaballa, M. Abbod, A. Jameel, N. Khaled, “Throughput maximization & power optimization analysis in non-orthogonal multiple access system,” in 2021 IEEE 4th 5G World Forum (5GWF), 2021, pp. 82–87, doi: https://doi.org/10.1109/5GWF52925.2021.00022.
Z. Yang, W. Xu, C. Pan, Y. Pan, M. Chen, “On the optimality of power allocation for NOMA downlinks with individual QoS constraints,” IEEE Commun. Lett., vol. 21, no. 7, pp. 1649–1652, 2017, doi: https://doi.org/10.1109/LCOMM.2017.2689763.
S. Boyd, L. Vandenberghe, Convex Optimization. Cambridge, UK: Cambridge University Press, 2004.
M. Gaballa, M. Abbod, M. Albasman, “Power allocation & MRC analysis for single input multi output non-orthogonal multiple access system,” in 2021 IEEE International Conferences on Internet of Things (iThings) and IEEE Green Computing & Communications (GreenCom) and IEEE Cyber, Physical & Social Computing (CPSCom) and IEEE Smart Data (SmartData) and IEEE Congress on Cybermatics (Cybermatics), 2021, pp. 168–173, doi: https://doi.org/10.1109/iThings-GreenCom-CPSCom-SmartData-Cybermatics53846.2021.00038.
M. Chen, R. Furness, R. Gupta, S. Puchala, W. (Grace) Guo, “Hierarchical RNN-based framework for throughput prediction in automotive production systems,” Int. J. Prod. Res., vol. 62, no. 5, pp. 1699–1714, 2024, doi: https://doi.org/10.1080/00207543.2023.2199438.
S. Ghimire, R. C. Deo, H. Wang, M. S. Al-Musaylh, D. Casillas-Pérez, S. Salcedo-Sanz, “Stacked LSTM sequence-to-sequence autoencoder with feature selection for daily solar radiation prediction: A review and new modeling results,” Energies, vol. 15, no. 3, p. 1061, 2022, doi: https://doi.org/10.3390/en15031061.
J. Zhang, Y. Jiang, S. Wu, X. Li, H. Luo, S. Yin, “Prediction of remaining useful life based on bidirectional gated recurrent unit with temporal self-attention mechanism,” Reliab. Eng. Syst. Saf., vol. 221, p. 108297, 2022, doi: https://doi.org/10.1016/j.ress.2021.108297.
Z. Qin, H. Ye, G. Y. Li, B.-H. F. Juang, “Deep learning in physical layer communications,” IEEE Wirel. Commun., vol. 26, no. 2, pp. 93–99, 2019, doi: https://doi.org/10.1109/MWC.2019.1800601.
Y. Wang, R. Zhang, L. Yan, X. Ma, “Pilot chirp-assisted OCDM communications over time-varying channels,” IEEE Wirel. Commun. Lett., vol. 12, no. 9, pp. 1578–1582, 2023, doi: https://doi.org/10.1109/LWC.2023.3283672.
A. S. M. Mohammed, A. I. A. Taman, A. M. Hassan, A. Zekry, “Deep learning channel estimation for OFDM 5G systems with different channel models,” Wirel. Pers. Commun., vol. 128, no. 4, pp. 2891–2912, 2023, doi: https://doi.org/10.1007/s11277-022-10077-6.
A. Sherstinsky, “Fundamentals of recurrent neural network (RNN) and long short-term memory (LSTM) network,” Phys. D Nonlinear Phenom., vol. 404, p. 132306, 2020, doi: https://doi.org/10.1016/j.physd.2019.132306.
H. Kim, Y. Jiang, S. Kannan, S. Oh, P. Viswanath, “Deepcode: Feedback codes via deep learning,” IEEE J. Sel. Areas Inf. Theory, vol. 1, no. 1, pp. 194–206, 2020, doi: https://doi.org/10.1109/JSAIT.2020.2986752.
M. B. Mashhadi, D. Gunduz, A. Perotti, B. M. Popovic, “DRF codes: Deep SNR-robust feedback codes,” ITU J. Futur. Evol. Technol., vol. 4, no. 3, pp. 447–460, 2023, doi: https://doi.org/10.52953/DAPE6014.
Z. Ding, Z. Yang, P. Fan, H. V. Poor, “On the performance of non-orthogonal multiple access in 5G systems with randomly deployed users,” IEEE Signal Process. Lett., vol. 21, no. 12, pp. 1501–1505, 2014, doi: https://doi.org/10.1109/LSP.2014.2343971.
A. Simonetto, E. Dall’Anese, S. Paternain, G. Leus, G. B. Giannakis, “Time-varying convex optimization: Time-structured algorithms and applications,” Proc. IEEE, vol. 108, no. 11, pp. 2032–2048, 2020, doi: https://doi.org/10.1109/JPROC.2020.3003156.
L.-T. Tu et al., “Performance analysis of multihop full-duplex NOMA systems with imperfect interference cancellation and near-field path-loss,” Sensors, vol. 23, no. 1, p. 524, 2023, doi: https://doi.org/10.3390/s23010524.
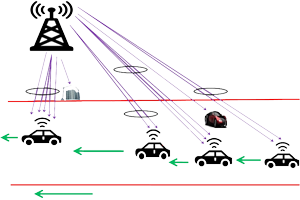
##submission.downloads##
Опубліковано
Як цитувати
Номер
Розділ
Ліцензія
Авторське право (c) 2024 Вісті вищих учбових закладів. РадіоелектронікаИздатель журнала Известия высших учебных заведений. Радиоэлектроника (сокр. "Известия вузов. Радиоэлектроника"), Национальный технический университет Украины "Киевский политехнический институт", учитывает, что доступ автора к его статье является важным как для самого автора, так и для спонсоров его исследований. Мы представлены в базе издателей SHERPA/RoMEO как зеленый издатель (green publisher), что позволяет автору выполнять самоархивирование своей статьи. Однако важно, чтобы каждая из сторон четко понимала свои права. Просьба более детально ознакомиться с Политикой самоархивирования нашего журнала.
Политика оплаченного открытого доступа POA (paid open access), принятая в журнале, позволяет автору выполнить все необходимые требования по открытому доступу к своей статье, которые выдвигаются институтом, правительством или фондом при выделении финансирования. Просьба более детально ознакомиться с политикой оплаченного открытого доступа нашего журнала (см. отдельно).
Варианты доступа к статье:
1. Статья в открытом доступе POA (paid open access)
В этом случае права автора определяются лицензией CC BY (Creative Commons Attribution).
2. Статья с последующим доступом по подписке
В этом случае права автора определяются авторским договором, приведенным далее.
- Автор (каждый соавтор) уступает Издателю журнала «Известия высших учебных заведений. Радиоэлектроника» НТУУ «КПИ» на срок действия авторского права эксклюзивные права на материалы статьи, в том числе право на публикацию данной статьи издательством Аллертон Пресс, США (Allerton Press) на английском языке в журнале «Radioelectronics and Communications Systems». Передача авторского права охватывает исключительное право на воспроизведение и распространение статьи, включая оттиски, переводы, фото воспроизведения, микроформы, электронные формы (он- и оффлайн), или любые иные подобные формы воспроизведения, а также право издателя на сублицензирование третьим лицам по своему усмотрению без дополнительных консультаций с автором. При этом журнал придерживается Политики конфиденциальности.
- Передача прав включает право на обработку формы представления материалов с помощью компьютерных программам и систем (баз данных) для их использования и воспроизводства, публикации и распространения в электронном формате и внедрения в системы поиска (базы данных).
- Воспроизведение, размещение, передача или иное распространение или использование материалов, содержащихся в статье должно сопровождаться ссылкой на Журнал и упоминанием Издателя, а именно: название статьи, имя автора (соавторов), название журнала, номер тома, номер выпуска, копирайт авторов и издателя "© Национальный технический университет Украины "Киевский политехнический институт"; © автор(ы)".
- Автор (каждый соавтор) материалов сохраняет все права собственника материалов, включая патентные права на любые процессы, способы или методы и др., а также права на товарные знаки.
- Издатель разрешает автору (каждому соавтору) материалов следующее:
- Право пользоваться печатными или электронными вариантами материалов статьи в форме и содержании, принятыми Издателем для публикации в Журнале. Подробнее см. политики Оплаченного открытого доступа, подписки и самоархивирования.
- Право бесплатно копировать или передавать коллегам копию напечатанной статьи целиком или частично для их личного или профессионального использования, для продвижения академических или научных исследований или для учебного процесса или других информационных целей, не связанных с коммерческими целями.
- Право использовать материалы из опубликованной статьи в написанной автором (соавторами) книге, монографии, учебнике, учебном пособии и других научных и научно-популярных изданиях.
- Право использовать отдельные рисунки или таблицы и отрывки текста из материалов в собственных целях обучения или для включения их в другую работу, которая печатается (в печатном или электронном формате) третьей стороной, или для представления в электронном формате во внутренние компьютерные сети или на внешние сайты автора (соавторов).
- Автор (соавторы) соглашаются, что каждая копия материалов или любая ее часть, распространенная или размещенная ими в печатном или электронном формате, будет содержать указание на авторское право, предусмотренное в Журнале и полную ссылку на Журнал Издателя.
- Автор (соавторы) гарантирует, что материалы являются оригинальной работой и представлены впервые на рассмотрение только в этом Журнале и ранее не публиковались. Если материалы написаны совместно с соавторами, автор гарантирует, что проинформировал их относительно условий публикации материалов и получил их подписи или письменное разрешение подписываться от их имени.
- Если в материалы включаются отрывки из работ или имеются указания на работы, которые охраняются авторским правом и принадлежат третьей стороне, то автору необходимо получить разрешение владельца авторских прав на использование таких материалов в первом случае и сделать ссылку на первоисточник во втором.
- Автор гарантирует, что материалы не содержат клеветнических высказываний и не посягают на права (включая без ограничений авторское право, права на патент или торговую марку) других лиц и не содержат материалы или инструкции, которые могут причинить вред или ущерб третьим лицам. Автор (каждый соавтор) гарантирует, что их публикация не приведет к разглашению секретных или конфиденциальных сведений (включая государственную тайну). Подтверждением этого является Экспертное заключение (см. перечень документов в Правила для авторов).
- Издатель обязуется опубликовать материалы в случае получения статьей положительного решения редколлегии о публикации на основании внешнего рецензирования (см. Политика рецензирования).
- В случае публикации статьи на английском языке в журнале «Radioelectronics and Communications Systems» (Издатель: Аллертон Пресс, США, распространитель Springer) автору (соавторам) выплачивается гонорар после выхода последнего номера журнала года, в котором опубликована данная статья.
- Документ Согласие на публикацию, который подают русскоязычные авторы при подаче статьи в редакцию, является краткой формой данного договора, в котором изложены все ключевые моменты настоящего договора и наличие которого подтверждает согласие автора (соавторов) с ним. Аналогичным документом для англоязычных авторов является Copyright Transfer Agreement (CTA), предоставляемый издательством Allerton Press.
- Настоящий Договор вступает в силу в момент принятия статьи к публикации. Если материалы не принимаются к публикации или до публикации в журнале автор (авторы) отозвал работу, настоящий Договор не приобретает (теряет) силу.